Enterprise Adoption of Generative AI
Generative AI has emerged as a significant force in recent years, poised to revolutionise how businesses operate, with many acknowledging its transformative potential in generating high-quality text, analysis, code, images, videos, and more from text prompts. In fact, a recent IBM survey revealed that 64% of CEOs feel pressured by stakeholders like investors and lenders to speed up the adoption of generative AI. But is the hype living up to adoption rates?
In this article, we will explore the realm of enterprise adoption for generative AI. We will delve into the challenges, current applications within organisations, barriers to adoption, and the future prospects of generative AI.

Who is using generative AI?
The adoption of Generative AI is increasing as companies incorporate it into their daily activities. GBK research found that a large number of business leaders have embraced Generative AI, with 37% using it on a weekly basis. Additionally, 81% of these leaders have formed specialised teams of 10 or more members dedicated solely to developing Generative AI strategies.
What kind of companies are at the forefront of adoption? Perhaps unsurprisingly, it is tech organisations leading the race which are likely best placed for adoption due to their culture, positioning and agility, and natural caution of avoiding the pitfalls of implementing enterprise generative AI. Implementation in the tech sector may have an easier time due to:
- Robust technological infrastructure: For instance, physical hardware for running complex algorithms, or cloud-based platforms for scalable processing and data storage.
- Presence of in-house technical expertise: Tech companies will likely have better in house expertise for technologies such as machine learning and natural language processing.
- Organisational agility and innovative culture: Tech organisations are typically structured for rapid experimentation and iteration and foster an environment that encourages exploration of new technologies.
- Technology stack: Technology companies often already leverage AI-driven automation, personalisation, and predictive analytics to gain a competitive advantage, making the adoption of generative AI a logical progression.

How are organisations using and deploying generative AI
The main drivers for implementing generative AI involve improving employee productivity, streamlining business processes, fostering employee innovation, and creating new products and services. Generative AI isn't yet a widespread major strategic initiative, but organisations are utilising it in various ways, for example:
- Marketing content: From copy, images, blogs, marketing emails, translation, to video, generative AI is employed to enhance content creation processes.
- Coding: Programmers provide plain text descriptions of their desired code.
- Data analysis: Generative AI provides proactive insights and uncovers hidden patterns in data, making analysis intuitive and generating easy-to-understand insights.
- Customer support: Generative AI can help understand customer intent, driving new customer service applications such as generating customer responses.
- Summarisation: Generative AI has the ability to generate summaries that are more engaging, informative, and unique compared to extractive methods, utilising synonyms and paraphrases.
- Research: Generative AI tools can enhance and optimise research, from discovering articles to composing literature reviews.
How is generative AI being deployed?
- Open source: Open source models involve setting up infrastructure but offer extensive customisation options. They are generally more cost-effective but may demand greater expertise to tailor them to your organisation effectively.
- Closed source: Major tech companies typically provide closed source models, offering pre-built generative AI solutions based on cloud infrastructure. While using closed source models is convenient and efficient, it can involve substantial costs.
- Generative AI as-a-service: Outsourcing generative AI development and deployment to a service provider provides tailored solutions and expertise that your organisation may lack. However, costs can escalate based on the provider's requirements, and there's a risk of overreliance on the supplier.
- Case specific generative AI technology: The generative AI field offers solutions that enhance specific operations such as data analysis or email marketing. While some solutions may be cost-effective initially, they can be challenging to scale and may lead to technical debt.
- In-house generative AI: Developing custom solutions in-house offers full control but demands rare and expensive skills. The associated costs of computing architecture and model training can quickly escalate. Opting for third-party solutions is often a more practical choice.
What are the risks and how to overcome them?
Although the hype and attention around generative AI have been significant, its adoption has not matched the same pace. This could be attributed to various factors such as complexity and cost, to concerns around data privacy and also a lack of understanding or company wide buy in. Here are the most common concerns enterprises face when adopting generative AI.
- Use case: Businesses face challenges in leveraging generative AI effectively, struggling to align AI strategies with business goals. Without a clear plan, integrating AI technologies into workflows and measuring ROI can be problematic, leading to inefficiencies and underutilisation of resources.
- Potential legal issues and compliance: Generative AI faces potential legal issues and compliance challenges related to copyright, data privacy, and ethical considerations.
- Company culture: Organisations may not have the enthusiasm to implement generative Ai technologies in a transformative way. Demonstrating the return on investment in generative AI can be challenging. The issue is further complicated by the absence of dedicated teams for AI initiatives and unclear leadership within IT and data science teams.
- Lack of quality data: For precise outcomes, the AI model requires access to an extensive array of data, including sales figures, market attributes, customer profiles, product information, and other relevant data sources.
- Cost: The expense of integrating generative AI into a company's operations can indeed be a major challenge. The initial costs include not only the technology but also the infrastructure required to support it. API expenses, hardware, data storage, deployment, and cloud computing costs can quickly deplete a budget.
- Expertise: The pool of experts in generative AI and machine learning is still limited, as these specialists are in high demand and quickly hired, resulting in a shortage of skills needed to drive generative AI implementation.
Key tips for implementing generative AI
Businesses can overcome common generative AI pitfalls by following essential tips to navigate the complexities of adoption and maximise its transformative potential.
- Obtain quality data: When using generative AI, limit training data to relevant information to reduce bias and protect sensitive data. Appointing a chief analytics or digital officer can improve understanding of AI applications, emphasise data management, and ensure accuracy. Early privacy measures protect data, while AI in some industries improves results by identifying personal data and enhancing datasets.
- Use data relevant to your use case: When it comes to implementing a significant change, such as generative AI, it's important to first identify the challenges and understand why you need it. This will allow you to create a strategy and establish a clear vision for the type of outputs you wish to generate, and how generative AI can be integrated into the process.
- Chip vs cloud: Cloud-based AI provides flexibility and accessibility, while chip-based AI offers faster processing and localised security. Choosing between them depends on budget, data sensitivity, and real-time processing needs. The cloud is commonly chosen for generative AI due to accessibility, while chip-based solutions may be more feasible for larger corporations with in-house capabilities. However, new suppliers offering cost-effective AI-enabled chips may alter market dynamics in the future.
- Understand roles and talent: Successful generative AI projects depend on forming a diverse team with proficiency in different areas, such as AI research, data science, domain knowledge, and software engineering. Collaboration and clear communication within the team are crucial to fully utilise the capabilities of generative AI technologies.
- Create an incident response plan: Protecting customer and company data is paramount. Take steps to avoid misuse of sensitive data, like feeding it into generative AI. Even though generative technology is becoming more common, it remains unfamiliar territory, so readiness for potential incidents is essential. Establishing an incident response strategy is critical to address any issues that may surface, including data breaches, signs of data bias, or the dissemination of inaccurate information.
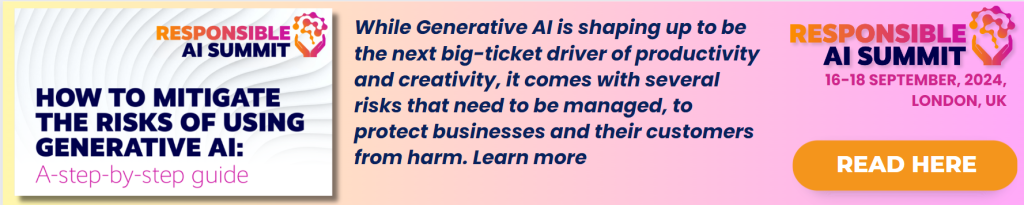
The future of generative AI
Around 42% of large organisations are currently implementing AI, and 59% of initial users are intending to broaden its application, according to IBM's research. The future of generative AI appears promising as major tech players such as Google, Microsoft, Amazon, among others, introduce their own AI solutions to the market fuelling innovation. Here are some trends we might be able to expect in the coming years.
- Multi-use generative AI: Generative AI may soon become an indispensable tool for office productivity. Rather than being labelled as AI, it will seamlessly blend into everyday software suites like Microsoft Office, Google Docs and Adobe tools, enhancing their capabilities.
- Optimised workflows: Startups are ready to transform workflows by introducing closed-loop systems that enable company-wide feedback and workflow enhancement. This fresh approach differs from traditional enterprise workflows, which typically include a disconnected combination of software, data systems, and human input.
- Reasoning abilities: The rise of sophisticated methods like agent architectures and chain-of-thought will enable organisations to participate in complex multi-step reasoning processes. This tackles a common limitation of LLMs, which is their difficulty with logical reasoning. While LLMs excel at generating logical and coherent text, their primary function is not to provide factual information or resolve issues.
- Utilisation of proprietary data: The next wave of AI-native technologies will possess the capacity to leverage and learn from proprietary data sets, including valuable customer feedback. Organisations will be able to develop industry-specific AI models by and also benefit from greater control over their data, allowing them to manage data access and usage effectively.
What next? Prepare your organisation for the generative AI revolution
If you want to keep on top of the generative AI landscape, mitigate its associated risk and adhere to new regulation? Register to attend the Responsible AI Summit 2024. This event brings together industry leaders, academia, and policymakers to provide actionable insights into the ever-changing realm of responsible AI, governance, and risk management. Its goal is to gain competitive edges, instill user confidence, and preemptively meet regulatory standards. Themes include:
- Promoting Responsible AI Practices in Enterprises: Exploring the Essential Evolution of Ethical and Compliant AI Deployment.
- Journeying Through AI Governance: Design, Deployment, and Monitoring for Continuous Advancement of Responsible AI Operations.
- Ensuring Quality Management in Generative AI: Maintaining Reputation, Values, and Impact.
- Establishing and Navigating an AI Ethics Council: Guiding the Future of Ethical AI Practices within Organizations.
Return to Home