In Conversation With the Founder of The Biometrics Institute
We spoke to Ted Dunstone of some of the wider lessons AI can learn from decades of experience in biometrics.
Add bookmark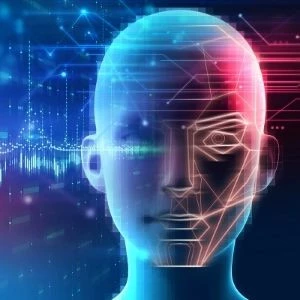
The below is an extract from a conversation from our 'Rise of Biometric Data' event, the first in our 'The AI, Data & Analytics Network Presents' series. In this conversation, we explored the entrepreneurial and ethical considerations of biometrics data with founder of the Biometrics Institute, Ted Dunstone. Follow the link to access the full conversation.
Elliot Leavy: The first question I would ask is, what do we mean by biometrics?
Ted Dunstone: As somebody who has been involved in biometrics from the very early days of its development, the story is quite an incredible one. Back when I began my PhD in 1992, biometrics was still a very obscure field, and, if anyone had heard about it at all, it was through science fiction. Fast forward to today, and it is found in so many different places across so many different applications, and almost everyone interacts with biometrics in some part of their daily lives now.
So, what do we mean by biometrics? Biometrics is the use of biological attributes to determine and identity related attributes. This can be in a smart gate context like at airports or it could be the phone that uses touch or facial identification to unlock.
Other common use cases include banking apps that require you to do any number of different types of biometric authentication, whether it's with your fingerprint, your face, even your voice.
Basically, anything which is distinctive about you, from a biological perspective, can be used as a form of what we call binding for identity processes, is a form of biometric.
And so, we're seeing the use of this and wider and wider types of contexts. But it doesn't necessarily only relate to things that require identification. There are some applications where it's used for instance for marketing and for determining the demographic information of an individual and then inferring from that information more appropriate services.
That’s a kind of a quick snapshot of biometrics, with the main biometric being fingerprints, face, speech, or voice.
Elliot Leavy: There are many more though aren’t there?
Indeed, there is iris recognition and even the reading of palm veins ‑ where they read the pattern of veins using infrared in the in the hand. Then there are even lesser-known ones such as behavioral, which can identify someone based on their personal typing dynamics and the analysis of the inter-key spacing can be used in determining or verifying identity.
Elliot Leavy: The ubiquity of this technology is seemingly endless. From cars shutting down when they realize that the driver is falling asleep at the wheel, to teachers being shown if their pupils are paying attention or not. Even call centers are listening to see how customers are responding emotional to the calls. What other sort of business practices might people not really be thinking about in the space when they when they when they think of biometrics?
Ted Dunstone: Another example would be anything where you are delivering an electronic service remotely. Nowadays, in banking, biometrics negates the need to have to go into a bank physically to set up an account in person. By identifying the new customer remotely and accurately, it can ensure that remote onboarding is trusted and safe.
Elliot Leavy: The key term there is trust. Since you've started back in biometrics, what has happened to the trust gap? Has it narrowed?
Ted Dunstone: I wouldn't necessarily say that the trust gap has narrowed, but I do think that use cases have exploded. This means there is an ever ongoing need to reinforce trust in the industry. Fortunately, there are some recent developments that have helped in that process, with open-source algorithms that are available which are much more robust than algorithms available only five years ago, that are better understood and can be better trusted as a result.
The overall quality and accuracy of systems has increased massively and the reason for that is advances in AI around deep learning and around building networks that can do this sort of matching at scale. By using these new deep learning techniques trust has been increased because the performance of the algorithms is now at a point where they can be deployed in lots of different ways and in ways that work.
But there is a lot of mistrust associated with biometric systems that stems from a misunderstanding about how data is handled, and further distrust comes in the form of a hangover from the black box problems associated with algorithms today.
However, one of the big developments over recent years is the creation of laboratories such as Bixie lab that can take biometric matching algorithms and provide internationally accredited certification for those systems.
Elliot Leavy: How do you create a worldwide framework for that? Or is there sort of different regions in the world who are doing it very differently now?
Ted Dunstone: There are several parts to this answer. The first part is the ISO standard. So the ISO standards are used in a whole range of different contexts for a whole range of different purposes to provide an internationally standard benchmark against which products can be tested, and accredited.
In biometrics case, two main components are tested. The first is accuracy – does it work? Another is what is called presentation attack detection – if I found a photo of you online, could I game the system with it or not?
Both of those standards are internationally recognized and can be tested and accredited in a way that allows them to be used in any jurisdiction. But the national, these trust frameworks, of which those standardized testing is just a part.
However, this space is still divided regionally. Obviously, the EU is doing one thing, the UK another and as well as both Australia and Singapore so this is still very much a work in progress.
Elliot Leavy: How easily are these systems vulnerable to hacking? I suppose we can even talk about deep fakes in that regard, with algorithms being trained on synthetic biometric data sets.
Ted Dunstone: No matter what area of security you happen to be working in, there's always an adversarial force right? People will come up with new techniques and new ways of trying to bypass the system. And then it's important that system designers have techniques that can reduce the risk. And you can reduce the risk in several ways reducing the risk doesn't always mean that you need to have a technological solution – it can be a process that hinders the potential for the attacker to succeed for example.
Addressing vulnerabilities is important, but these are not vulnerabilities that are only specific to biometrics, but that underpin all then AI industry in general. All models make implicit assumptions about the data it was trained under, and if you have a malicious actor that is looking to game the system or change the system in some way to their advantage, there will always be ways to attempt that.
Elliot Leavy: This goes back to our previous conversation, about what lessons the wider AI space can take from biometrics and vice versa.
Ted Dunstone: Yes, and one area that the wider industry can learn from biometrics is accountability and oversight because biometric systems deal with sensitive personal data and, just like many other AI systems, might be making decisions based on people's that have significant impacts.
What this really comes down to is governance. The governance framework needs to take into account how the model has been tuned, what the adverse outcomes of that are etc. Just like any AI systems, biometric systems should never be left unattended and without some sort of monitorial oversight.
There is always necessary a degree of human oversight over how our model is performing, both to check on adverse outcomes, not only for ethical reasons but also functionary ones as contexts change over time. In a biometric sense, how sensors work can change radically in different times of year for example. If you are not monitoring that, people’s lives can be affected by the lack of governance at work.
Elliot Leavy: What are the other areas where lessons can be drawn from biometrics?
Ted Dunstone: There’s quite a few in fact, from privacy and the protection of data, those who have worked in the biometrics space for as long as I have have seen it all. Explainability – a hot topic right now – has been key to any biometrics system since…
To listen to the rest of the conversation, just follow the link to our ‘Rise of Biometric Data’ talk now.