5 Things to Know About Data Curation
A Mini-Guide to Data Curation and What it Means for the Data-Centric Enterprise
Add bookmark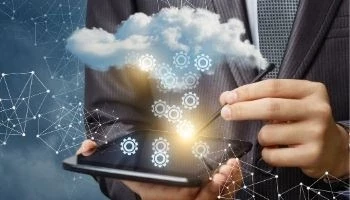
What is Data Curation?
As defined by tech republic, data curation is “the art of maintaining the value of data.” It is the process of collecting, organizing, labeling, cleaning, enhancing and preserving data for use. The goal is to ensure data is “cared for” throughout its lifecycle so that its FAIR (Findable, Accessible, Interoperable, and Reusable) and one can derive as much value from it as possible.
Common data curation activities include:
- Contextualizing- Using metadata to link the data set to related sources and attributions and/or projects that provide added context for how the data were generated and why
- Citing the Data - Adding citations to support appropriate attribution by third-party users in order to formally incorporate data reuse
- De Identification - Redacting or removing personally identifiable or protected information
- Validating and Adding Metadata - Information about a data set that is structured (often in machine-readable format) for purposes of search and retrieval.
- Validation of Data- The review of a data set by an expert with similar credentials and subject knowledge as the data creator to validate the accuracy of the data
Why is data curation important?
As business intelligence and advanced analytics emerge as key enablers of enhanced strategic decision making, data has evolved from an ancillary byproduct of business operations into a powerful strategic asset.
In addition, many legacy companies who may have lacked proper governance frameworks in the past, sometimes find themselves stuck in a “data swamp.” Data swamps happen when data lakes - receptacles for storing and accessing data - are not properly maintained. As a result, valuable data gets lost in a sea of corrupt or unusable data.
However, data curation helps prevent this by ensuring data is organized, described, cleaned, enhanced, and preserved before it enters the data lake. Furthermore, data curation techniques can be applied to data swamps to help restore them back to data lakes.
Last but certainly not least, effective data curation is especially important for ensuring machine learning (ML) and artificial intelligence (AI) training data is primed for processing. In other words, that data is machine readable, reliable and unbiased. By ensuring data is properly labeled and categorized, data curation can help data scientists and AI developers validate the diversity of training data.
Data Curation vs. Data Governance
Simply put, data governance is a business strategy and, conversely, data curation is an iterative process. While data governance outlines the roles, process, and policies that control data management practices, data curation is chiefly concerned with optimizing metadata so that data is easily discoverable, achievable and preservable.
That being said, data curation and data governance are intrinsically intertwined. In fact, data curation is a component of successful data governance strategies.
Data Curation Challenges
Data curation can be an incredibly arduous process as well as a very expensive one, especially when it comes to curating massive volumes of unstructured data for “big data” usage. In this scenario, multiple data curation approaches are often needed to properly sort through and manage high volumes of diverse data sets.
In addition, for decades, companies have hoarded data away with little consideration regarding what they intend to use it for and how to best protect it from decay. Though many organizations may want to use this data, they have no idea where to start and lack a sound enterprise data strategy to lead the way forward. Before data curation can take place, organizations must have a clear understanding of what types of data deliver the most value, why and how it can be used in order to ensure success.
Data Curation Tools
Data curation platforms such as Alation, Stitch Data (Talend), DQLabs and Alteryx, optimize the pre-processing stages of the data management lifecycle to help ensure data integrity and usability. Using AI and ML, these tools validate metadata and organize insights into the correct repository.