A Look at ASOS’s Fashionable Approach to Data Science
How ASOS is reinventing the digital retail experience with predictive analytics and personalization
Add bookmark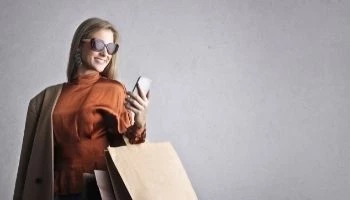
E-Commerce giant ASOS is setting trends in more ways than one. In addition to becoming the go-to shopping destination for the TikTok generation, ASOS is also making waves when it comes to data science and innovation.
As many retail companies have struggled to navigate the COVID-19 crisis, ASOS reported a 329% increase in before-tax profits and a 19% increase in global retail sales as its final year results for 2020.
The secret to its success: a focus on the customer experience and innovation. Like many of the other retailers we’ve covered such as Nike and Zappos, ASOS is leveraging advanced analytics and artificial intelligence (AI) to not only adapt to changing consumer habits but redefine the customer experience.
AI-Powered Personalization
Connecting shoppers with relevant products is paramount to the success of any retail business. In order to help its 24 million customers more effectively and efficiently navigate its catalogue of over 85,000 products, ASOS turned to AI and predictive analytics.
Taking a step back, at the core of ASOS’s personalization strategy lies a deep understanding of its products. Using deep neural networks ASOS is able to classify products (both the image and description) based on certain attributes such as item type, color, print, style, length, occasion, etc.
*Image sourced from “Deep learning for fashion attributes” https://medium.com/asos-techblog/deep-learning-for-fashion-attributes-763c8c95034c
The second component is a deep understanding of how customers interact with its products. As explained on ASOS’s tech blog, “The rationale is that, if two products are purchased by the same customers often, they are similar in some way. The set of algorithmic approaches relying on this type of customer-product interaction are known as collaborative filtering and have proven to be a very powerful way of creating personalised recommendations.”
For new products that lack such data, ASOS created a “customised content-aware model that is able to leverage customer-product interactions together with the augmented product attributes. This hybrid approach incorporates several state-of-the-art advances in recommender systems and not only incorporates new products, but also enhances the recommendations that customers receive overall.”
Another example of data-driven personalization at ASOS is its brand recommendations API. Run on a constellation of Azure Machine Learning solutions including Deep Learning Virtual Machine, the ML-powered tool provides customers with a personalized list of brands based on their past browsing and purchasing behavior.
In 2020, ASOS took their personalization approach to the next level by developing an outfit recommendation tool. Instead of simply recommending similar items, the outfit generation tool seeks to recommend complementary items - a much more complicated task.
In a nutshell, the tool consists of a “multilayer neural network fed by visual and textual features to learn embeddings of items in a latent style space such that compatible items of different types are embedded close to one another.”
In other words, it analyzes and groups together items based on attributes. For example, if someone is viewing the product page of a red ball gown, in the recommended products section below the primary image they might see matching high heels, evening bags and jewelry.
The Future
Fashion consumers are notoriously fickle and what’s in one day is out the next. Though ASOS has yet to develop a predictive algorithm capable of predicting the next big trend, they’re at least developed a reliable and effective way of upselling consumers based on their immediate priorities.
As the company continues to grow globally, integrate new brands and expand its marketplace platform, it will be interesting to see where and how data-fueled innovation fits in.
Become a Member of the AI, Data & Analytics Network TODAY!