Increasing the Quotient for Data Science within the Leadership Team
A conversation with Miao Song, CIO, GLC
Add bookmark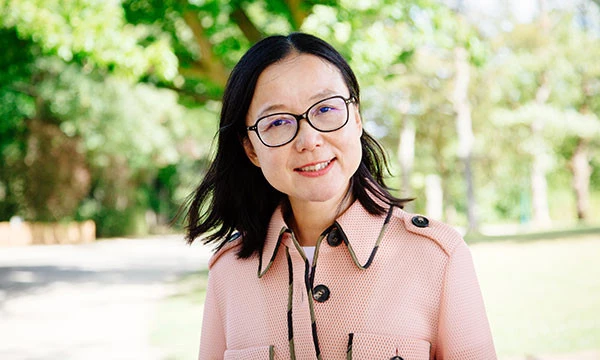
Transitioning artificial intelligence out of the lab and into the real world can be incredibly challenging. However, the struggle doesn’t end there. Ensuring that AI-powered insights are actually used to drive transformational value is the second half of the battle.
With this in mind we invited Miao Song, the chief information officer of GLP, to share her tips for developing high impact AI initiatives and teams at the upcoming Applied AI APAC virtual event this May 3-4, 2022. While you stay tuned for more details on the event, here is a small snippet of what you can expect to hear from Miao during her session.
IAN: How do you go about procuring data science talent in such a tight market?
Miao Song:
Yeah, so to be honest, it's a little bit hard to find the data science talents in this industry because it's not just understanding data and technology, but also understanding the use cases, the art of possible, and really playing business insights into the work. So my strategy is to educate internal talent and, at the same time, bring in best practices as well as talent from the external world and even other industries.
So, I do both. I basically buy and build to make sure that the right people can work on these initiatives. I think we had a good starting point, but still, a lot of things to be done here which is to be expected. I think some companies are a little bit ahead in this area but we’re only just getting started and the potential is huge.
IAN: Ok, so let’s say our team is in place but we're not going to have success unless strategy is aligned? Translation from business to data science and from data science to business. How do you approach this challenge?
Miao Song:
The first thing to look at are use cases and what I call “the art of possible.” Those are the most important parts because if you only talk about data science, people don't really relate what you are doing with reality, so your AI project has to be insights driven and value driven.
We’ve collected a lot of data in the past few years around our operation. For example, with Smart Park, we collected IoT data, we collect traffic data, we collect some customer data to actually create value. Basically, the data is there, but there are not many insights from the work we did in the past. So, now we look at this very differently. We think about, Hey, think about the value. What's the value to the customer and how can we actually create value in our operation.
In the longer term, we’re looking at how this data can be used to predict new things such as industry trends. We actually think about traffic information because different customers are in different stages, right? For example, during COVID, even in the eCommerce space, different eCommerce customers may have different operations or different business growth. We use traffic information to predict the industry trend that is really beyond just operation.
IAN: Now, you can get into an area of not being able to distinguish between correlation and causation when you have that data in front of you. So how do you draw that distinction? What are the means to make sure that you don't get fooled by patterns?
Miao Song:
I think one is really looking at, first of all, the size of data, the volume of data, it's probably more data than you have, you have much better trend analysis and accuracy, but it's not just the only element. There are other elements that have to be considered.
So, for example, if you predict trends, there has to be some reality check. There has to be some interpretation of the output of that analytics and that work has to be done by the data scientist. They need to work alongside the business to figure out whether the insights make sense or not.