Quant Failures Highlight the Limitations of AI
Separating the hype of AI and machine learning from the reality
Add bookmark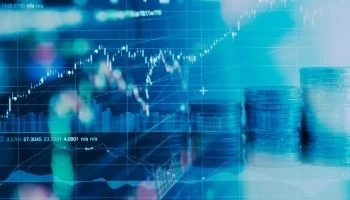
2020 was a wild ride for many investors. At the height of the pandemic in March 2020, the global stock market declined by over 30% and single-day stock market swings of 5% or more became a common occurrence.
Even now, in May of 2021, the recovery has been uneven. In between political turmoil, supply chain disruptions, cyber attacks, inflation and the continued threat of the Coronavirus, the turbulence is far from over.
Amidst this chaos, some investors reaped record revenues, others floundered such as, most notably for us, quant funds and others who rely on AI-powered trading models.
Quantitative funds or “quants” are investment management vehicles that rely on complex mathematical models, artificial intelligence (AI) and automated algorithms to make trading decisions. Though quants are not necessarily “smarter” or better at trading than their human-led counterpoints, they are significantly more efficient and, as they require less overhead (i.e. human traders), they are able to charge smaller fees.
However, this is where the problems arise.
Artificial intelligence (AI) and machine learning (ML) and associated solutions work by analyzing high volumes of historical data searching for patterns. As such the outputs created by the neural networks are only as good as the data being fed into them.
Market volatility - with its extreme, unpredictable fluctuations - wreak havoc on AI models. As Ernie Chan, managing member of QTS Capital Management, explained to Wired Magazine, “It’s easy to train a system to recognize cats in YouTube videos because there are millions of them. In contrast, You can count [these huge market swings] on one hand. So it’s not possible to use machine learning to learn from those signals.”
Though some quant firms were able to successfully navigate the pandemic year, a handful of high profile firms collapsed or came close to it. In fact, according to Aurum Fund Management, weighted by assets, the average quant hedge fund lost 5.7% by August 2020, compared with the average hedge fund’s 5.2g% gain.
So what does this mean for me?
Rest assured we’re not here to advise you on your investment portfolio. However the challenges quant funds faced over the last year highlight many of the limitations of modern day AI - chiefly that it’s brittle and data-dependent.
Companies have made big bets with AI and advanced analytics. However, due to a fundamental misunderstanding of what these tools can and cannot do, fail to transform these big ambitions into tangible value. As summed up by Anne Tucker, faculty director of the Legal Analytics and Innovation Initiative at the Georgia State University College of Law, AI leverages “components of human judgment at scale. It’s not a replacement; it’s a tool for increasing the scale and the speed.”
AI is a powerful tool for solving narrowly defined problems such as:
- text-to-voice translation
- identifying fraudulent credit card activity
- providing personalized recommendations and promotional offerings to customers
- monitoring and optimizing critical data center processes
- Predicting mortgage loan risk
However, just as AI won’t “crack the stock market,” it also won’t cure cancer or invent the next blockbuster product. It can only make the pursuit of such things more efficient and error-proof.
While using AI to enhance decision making and deliver real-world, tangible value can be incredibly challenging, organizations are making it happen. In fact, we have an entire virtual event devoted to the topic: Applied AI Live featuring AI masterminds from Credit Suisse, Northrup Grumman, Schlumberger and more.
Become a Member of the AI, Data & Analytics Network TODAY!