Maximizing the ROI of Customer Intelligence with AI
Add bookmark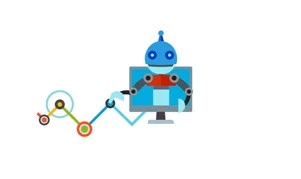
So much has changed in the customer insights realm over the past 30 years. Today we have more data, automation and predictive capabilities than ever before. Retail and CPG companies are taking full advantage of this moment and are starting to use customer data in new and exciting ways.
With this in mind, we invited Vincent de Stoecklin, Senior Solutions Architect at Dataiku, to share some of the most innovative and interesting use cases of customer intelligence analytics.
To break things down, Vincent explained that, generally speaking, there are 2 types of use cases: pre-sales (marketing, personalization and customer acquisition) and everything that happens afterwards (customer loyalty and engagement). The primary objectives of these two use cases are:
- How do you optimize the cost of new customer acquisition?
- How do you quantify the value of customer loyalty & retention (weighted lifetime value)?
The goal for most retailers and consumer product groups is to optimize the balance of acquisition vs. loyalty. As most business leaders know, retaining existing clients tends to be more cost-effective, but you still need new customers to drive growth. However, advanced analytics, such as the ones Dataiku enables, can shed light on the optimal balance. For example, below is a chart illustrating business value vs. complexity of customer intelligence initiatives:
From there he goes into 3 specific use cases. The first, a large European bank was looking for a data analytics platform that increased customer retention by anticipating churn and recommending relevant offers and services via marketing channels (i.e. email, website, in-branch, etc.)
In partnership with Dataiku, they were able to build a predictive system based on customer life events and usage patterns. Using that information, they were able to assign each customer a “churn score” and target them accordingly. For example, if someone with a high churn score walked into a bank, the system would notify the bank teller who could either offer them new services or help them adjust their banking options to better suit their current life goals.
By adopting this new, advanced customer churn prediction system, the bank was able to increase retention by 12% and lift, or accuracy of the predictive model, by 3X.
The 2nd example Vincent shared was for a B2B software vendor who wanted to build a real time recommendation engine. This new data-driven capability would accurately predict what individual shoppers would likely buy next, provide individualized retraining of models and include champion/challenger mode.
Working with Dataiku they were able to put such a system in place which ultimately increased average basket size by 7%
The 3rd “use case” or, rather, “a new way of working around consumer insights and marketing analytics,” focused on Unilever total data analytics reinvention. Tired of going through third party vendors and using massive market research campaigns that only provided incomplete, qualitative data, Unilever decided to partner with Dataiku to build a new, advanced, self-sustaining customer analytics system.
Unilever’s new, integrated system pulls insights from a wide range of sources (CRMs, social media, etc) and AI-powered automation tools transform that data into predictive insights. And, using next gen data visualization tools, they’re also able to effectively communicate these insights to the business. Using this new system, Unilever is able to democratize advanced customer analytics to deliver unprecedented business results across the business.
To close things up, Vincent offers 5 best practices for AI project results:
- Define process around project scoping and prioritization
- Think about the outcomes and include business from A-Z
- Explore and iterate to create relevant features
- Combine supervised and unsupervised approaches
- Deploy and operationalize your data flows in robust and governed way
Want to learn more? Watch the full Webinar On-Demand for FREE here!