Chief Data Officer, Harjot Singh’s Shares His 4 Step AI Framework
“If artificial intelligence is your penthouse, then your data management strategy is your foundation”
Add bookmark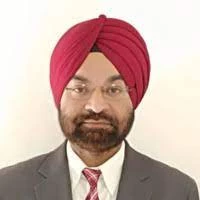
To say getting started with artificial intelligence (AI) isn’t easy could be the understatement of the century. In between extensive data infrastructure investments and technical requirements not to mention training, cultural transformation and strategic planning evolved, applied AI projects can be incredibly daunting.
However, luckily for us, AI expert and RAC WA’s Chief Data Officer, Harjot Singh recently shared his framework for AI implementation. Please keep in mind, the following is just a small sampling of what he will be sharing when he presents at the Applied AI virtual event taking place June 29-30 during his session on Data Is The Core: Applied AI For Business Resilience Through Customer-Centricity.
If artificial intelligence is your penthouse, then your data management strategy is your foundation
According the Harjot, applied AI requires 4 things:
- Robust data architecture and massive cloud storage capabilities
- Process redesign and automation
- Front-end facing app
- Service orchestration
According to Harjot, these four components build a “skeleton” whereby “all you have to do is plug in those products to your engine. And then you can start launching new products very quickly in the markets. And you get a first movers advantage. Then you have an obviously omni-channel interface strategy and then your product simplification and customization.”
READ NEXT: 5 Data Architecture Trends Shaping 2021
It all comes down to data quality and data governance
We can’t say it enough, AI success requires high quality data and extensive controls.
“What most people do wrong is that they start talking about machine learning and use cases, but without investing in the data quality or without investing in the governance process. What happens if you just realize that, yeah, your machine algorithm was not performing well, or it's not giving you accurate results because the sample data you took was not good enough or the training data you took was not good enough.”
Though there is certainly not a one size fits all approach to data governance, key considerations for establishing such frameworks are:
- data quality controls
- data architecture
- data modeling and design
- data storage and operations
- metadata management
- data security
- data integration and interoperability
- unstructured data management
- regulatory compliance
READ NEXT: AI Democratization Part Two: Data Governance as the Engine Powering AI Success
Don’t just automate processes, redesign them
All too often, organizations simply lift and shift processes, automating them with little thought as to how they could be optimized to enhance the customer experience. Not only is this a huge missed opportunity, it could also derail AI outcomes.
Process redesign helps ensure that backend and front end channels are fully connected. As Harjot explains, “people invest in the front and they've got a nice fancy app. They got a nice website, but it doesn't interact with their backend data. And most often this happens in legacy IT environments because they don't have a service orchestration layer or micro API based microservices architecture.”
He adds, “So you need to invest in a nice service orchestration platform or integration platform so that you can combine your front-end channel with the backend channel to give a better customer experience. So all this combined, I mean, these are just the basics of what, when we say that we start driving towards the digital transformation or AI based strategy.”