5 Data Architecture Trends Shaping 2021
Add bookmark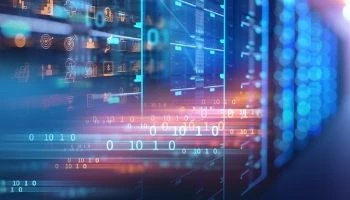
The Rise of DataOps
As defined by CIO Magazine, DataOps (data operations) is an agile, process-oriented methodology for developing and delivering analytics.The goal is to enable:
- Rapid innovation and experimentation delivering new insights to customers with increasing velocity
- Extremely high data quality and very low error rates
- Increased collaboration between the development team (the code creators) and the operations team (the code consumers)
As companies look to reap more value from data as well as usher in a new era of artificial intelligence (AI)-driven innovation, DataOps is proving to be a powerful way of achieving these objectives. In fact, over 10,000 people signed the The DataOps Manifesto. In addition, according to a 2020 study, Rethink Data: Put More of Your Business Data to Work— From Edge to Cloud, while only 10% of organizations report having implemented DataOps fully across the enterprise, a majority of respondents say that DataOps is “very” or “extremely” important.
Democratization of the Data Stack
If data-driven insights are the key to growth and innovation, it stands to reason that organizations would want to ensure that as many people as possible have access to them. As a result organizations are embracing tools such as data visualization and low-code tools to democratize data and analytics.
In fact, according to a study conducted by Google and the Harvard Business review, 97% of industry leaders surveyed said democratizing access to data and analytics across the organization is important to business success.
As we covered at our recent AI & Data Democratization event (which you can attend NOW on demand), organizations are developing new ways of providing non-technical employees with the analytics tools and knowledge to utilize and action enterprise data. For example, BNP Paribas built a state-of-the-art Enterprise Data Nerve Center to not only democratize data and therefore innovation, but ensure its business-led analytics project stayed compliant to the organization’s complex regulatory requirements.
AI Ready Architecture
Cutting-edge data science applications such as advanced analytics, applied AI and machine learning require high-volume and high-velocity data environments, a state that, at this point, can only be achieved with high performance data architecture models.
According to RedHat, An AI-ready, high performance data architecture model typically includes:
- AI/ML and DevOps tools
- Data pipelines provide cleaned data to data scientists for creating, training, and testing ML/DL models and to application developers for data management needs.
- A cloud platform gives data engineers, data scientists, ML engineers, and application developers access to the resources they need to work rapidly.
- Compute, storage, and network accelerators speed data preparation, model development, and inferencing tasks.
- Infrastructure endpoints provide resources across on-site, virtual, edge, and private, public, and hybrid cloud environments for all stages of AI/ML operations
*Image sourced from "A Unified Data Infrastructure Architecture," https://a16z.com/wp-content/uploads/2020/10/Data-Report-Martin-Inline-Graphics-R8-1.pdf
Back to the Basics - Data Governance and Quality
In between the race to embrace data-driven innovation and evolving data-related regulations, effective data governance and quality have never been more important. Though previously dismissed as a compliance issue in the past, data governance is increasingly becoming a strategic objective and a moral imperative.
In fact, according to a recent Teradata study, 77% of global business leaders say that their organizations are more focused on data accuracy than ever before. This is because not only do strong data governance and cleansing practices make data machine readable, it also helps combat AI bias.
Meet the Analytics Engineer
Traditionally speaking, data engineers were responsible for design data infrastructure while data scientists and analysts focused on using statistical modeling to analyze data. Now a new role is emerging: the analytics engineer.
The analytics engineer sits at the intersection of data scientists, analysts, and engineers, bridging the gap between the various teams. Both technically skilled and business-minded, it’s their job to build tools and infrastructure to support the efforts of the analytics and data team as a whole. At some companies they’re also responsible for democratizing data throughout the enterprise and incorporating self-service analytics into customer-facing products.
Become a Member of the AI, Data & Analytics Network TODAY!