5 Data Visualization & Storytelling Mistakes to Avoid
Instead of taming data, poorly designed visualizations can render data useless or, worse, lead to faulty decision making. Here are 5 ways that can happen.
Add bookmark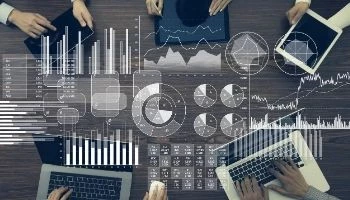
By making data-driven insights easy to share and understand, data visualization and storytelling are powerful tools for transforming data into action. However, with great power comes great responsibility.
Multiple studies over the years have proved that people believe what they see, especially if a chart is involved. On the other hand, charts and other visualizations that are poorly rendered can increase confusion as well as distrust. Furthermore, using data to inspire change requires more than just sharing numbers. These figures must be part of a bigger narrative that resonates with the hearts and minds of your intended audience.
With this in mind, here are a couple of ways data visualization and storytelling can go wrong.
#1 You Picked the Wrong Chart
Picking the wrong chart to display information is by and large the number one mistake people make. Though pie charts, for example, are perfect for communicating simple percentages, they should never have more than 5 “slices.” Howere, pie charts remain the most popular graph style in existence.
Selecting the right graph to display your information can be surprising challenging, however, using the wrong chart style will undoubtedly lead to confusion and mistrust. In addition to pie charts, some commonly misused types include:
- 3D charts.They look great but, because of the way our brains process them, they tend to misrepresent data.
- Bar Charts are useful for comparing parts of a bigger set of data, highlighting different categories, or showing change over time. However, they’re easy to overload. As a rule, bar graphs should have no more than 10 bars and are not appropriate for illustrating concepts that require multiple, connected data points. Instead, you should use line charts.
- World clouds are often used to communicate the insights derived from natural language processing (NLP) and AI Sentiment Analysis. However, in reality, they offer very little value as words without context mean nothing. Bar charts, treemaps and a host of other techniques are much more effective for conveying text-derived insights.
Which Image is Easier to Decipher?
VS.
#2 You Don’t Know Your Audience
Knowing your audience is critical for creating impactful data visualizations and democratizing data science in general. Not only does this help ensure you’re sharing the right data points, but that you’re also sharing them in the right way.
All too often, data storytellers overestimate or underestimate their audience. While a group of non-technical managers might be intimidated by technical jargon and big complex graphs, a group of IT managers might feel differently.
READ NEXT: The BI & Data Analytics Trends that will Define 2021 PART ONE
#3 You Don’t Get To Your Point
When it comes to using data to tell a story, providing context is critical. However, if the goal is to facilitate evidence-based decision making as quickly and effectively as possible, too much information will only confuse your audience.
When viewers are overwhelmed with data, their brains can’t process what they’re seeing and they tune out. If you can’t tell your data points apart or if it’s hard to read, chances are your visualization is overcrowded. One simple solution is to simply break the visualizations into smaller pieces.
READ NEXT: Make Data Accessible to Everyone with Data Democratization
#4 You Forget About Text & Context
Without context, data is meaningless. With that in mind, it’s surprising how often people forget or misuse text and labeling. No matter what you’re creating, whether it is a dashboard or a client presentation, every graph should be accurately labeled and titled.
For example, look at the graph below and try to guess what it’s trying to convey.
*Image sourced from "27 hilariously bad maps that explain nothing," https://www.vox.com/2015/2/18/8056325/bad-maps
Another common mistake when it comes to the language used to describe visualizations is unintentional bias. The text that accompanies visualizations is meant to give viewers objective context, not manipulate their perception of the data.
#5 You Use or Enable Flawed Data Science
Even seasoned data scientists can make statistical mistakes. For those less familiar with formal data science techniques, falling prey to statistical fallacies such as cherry picking, survivorship bias and false causality is incredibly easy. Poor design choices can exacerbate or even lead to data fallacies simply by misrepresenting reality, purposely on not.
To help you avoid that fate, here’s an infographic that outlines the most common data fallacies and how you can avoid them.
*Infographic sourced from https://www.geckoboard.com/best-practice/statistical-fallacies/
Become a Member of the AI, Data & Analytics Network TODAY!