Data Lakes vs. Data Warehouses
Both are powerful enterprise data and analytics tools. But what scenario is right for your organization?
Add bookmark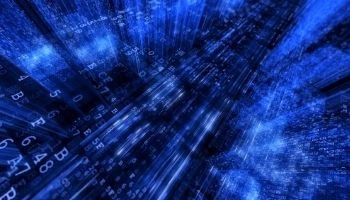
What is a data warehouse?
A data warehouse is a database that not only stores data, but processes and organizes it to ensure analytical readiness. Using a process known as extract, transform and load (ETL), data warehouses collect raw data from various sources, “transforms” it into structured data and then finally, loads it into the Data Warehouse system. Data that doesn’t answer concrete business questions is not included in the data warehouse, in order to reduce storage space and improve performance.
What is a data lake?
Data lakes are vast repositories of raw, unprocessed data the purpose of which is yet to be determined. Using data lakes, data scientists can freely explore and experiment with different data types of data sets. As they can be easily built and scaled without complex ETL processes, data lakes also offer organizations more agile and cost-effective data storage.
When in the hands of seasoned data scientists and engineers, data lakes can be powerful enablers of innovation as cutting edge technologies such as artificial intelligence and machine learning thrive on large, diverse data sets.
So which one should I use?
Though data lakes and data warehouses represent two types of data storage options, they are far from interchangeable. In fact, they’re more complementary than anything.
While data lakes allow for increased agility and free-style innovation, they are not without significant drawbacks. First and foremost, data lakes can easily turn into “data swamps” if they’re not properly maintained and governed. In other words, unlike data warehouses, data lakes do nothing to ensure that data is accurate and usable so they can’t be treated like data dumps. Without contextual metadata, the data stored in data lakes is undiscoverable and gets lost in the system. Effective data curation strategies are required to ensure data is usable and findable.
Though data lakes are often touted as enablers of data democratization, the average business user would probably struggle to make use of a data lake without extensive training. Access to data lakes must be paired with data literacy training in order to ensure business users are able to build and action their own data-driven reports and analytics products.
Furthermore, as the data stored in data lakes isn’t always accurate or verified, it could lead to faulty insights and analytics. Not only could potentially unreliable data cause a business user to make a bad strategic decision, it could also increase distrust between the data team and the business.
On the other hand, data warehouses are the ideal solution for producing standardized BI reports (i.e. weekly sales reports), dashboards and OLAP (online analytical processing). As data warehouses tend to be easier to use, they may be more suitable for non-technical users.
In a nutshell, data warehouses provide a centralized, single source of truth that can be used to enhance decision making. Whereas data lakes are an excellent data science tool for running AI/ML applications and high performance computing analytics (HPCA) as well as general experimentation.
Today most modern data architectures include both data warehouses and data lakes. For example, data can be first fed into a data lake where it’s“onboarded” and then, if applicable, funneled into the appropriate data warehouse for further processing.
Organizations are also developing hybrid solutions. For example, creating a data lake that verifies and filters out low quality or unsuitable data before ingestions. Or data warehouses that have embraced some of the more agile and flexible qualities of data lakes.