AI ROI: Calculating the Value of Machine Intelligence
Balancing the Near-Term and Long-Term Value of Artificial Intelligence
Add bookmark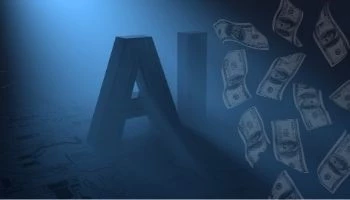
There are few assets companies value more than their data. However, data is an intangible asset - virtually impossible to quantify via traditional accounting methods and only of value when used strategically. For those organizations who collect and store data without ever applying it to decision making, data is actually a financial and regulatory liability.
When it comes to artificial intelligence (AI) these problems are magnified. AI is an experimental, iterative discipline where only a small percentage of AI models even make it into production, much less actually deliver tangible business outcomes. In fact, according to our own research, at most companies, less than 10% of AI models are ever used to enhance decision making.
With that in mind and given the expense of AI projects, most [if not all] organizations are years away from realizing true ROI on enterprise AI investments. However, that does not mean you cannot measure the success of AI projects or create evidence-driven business cases for AI.
Determining the Value of AI
Very generally speaking, there are two types of AI projects: ones that are likely to deliver near-term value and so-called moonshot projects - projects that have the potential to be transformational but require a substantial, long-term investment [and plenty of patience].
For short-term AI projects, ROI is typically centered around measurable things such as increased productivity, process efficiency and cost-savings. On the other hand, moonshot projects require the inclusion of more strategic, growth-oriented deliverables such as new revenue streams, market expansion and increased business per customer.
In industries where AI will likely play an outsized role in years to come (i.e. big tech), organizations are also incorporating the projected value of AI in 10+ years. This not only includes AI deliverables, but all of the assets associated with AI: data, talent, models, systems etc.
However, the value of AI shouldn’t be limited to quantitative metrics. Additional qualitative measures that one should consider addressing when determining the value of AI:
- Improved customer experience
- Skills and employee retention
- Increased competitive advantage
- Increased agility & operational resilience
- Enhanced brand reputation
- Improved accuracy and effectiveness
- Increased pricing power
Calculating the Cost of AI
Similar to determining value, measuring the cost of AI isn’t always so simple. To start, you have computing costs. This includes IT infrastructure, accelerator hardware such as GPUs and the energy required to power these systems. Secondly, you have the cost of acquiring, cleansing, processing, storing and managing large amounts of training data. Finally, you have employee compensation. Though timelines and resourcing needs vary, simple AI projects require at least one month and 3 employees (project manager, data engineer and data scientist) to properly execute.
In other words, compute + data + people = the cost of AI.
Though the cost of AI can vary wildly from roughly $40,000 for more basic AI initiatives (i.e. a small product personalization engine), larger, more complex enterprise AI projects can extend well into the millions. Given the high failure rate of AI, it’s easy to see how AI costs could easily spiral out of control.