The BI & Data Analytics Trends that will Define 2021 Part TWO
How Edge Computing, Data Monetization and AI will continue to shape the year ahead
Add bookmark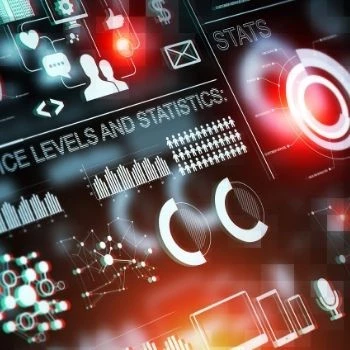
Last week we dug into 3 of the 6 BI & Data Analytics Trends that will Define 2021: Data Democratization, Streaming Analytics and Hyper-Personalization.
Today we look at the next 3.
Edge Computing
Simply put, edge computing (EC) is the practice of capturing, processing, and analyzing data near where it is created instead of sending the data to cloud data center. By decentralizing processing power, EC enables real-time processing without latency while reducing bandwidth and storage requirements on the network.
Edge computing is often used in industrial settings for predictive maintenance where IoT sensors can monitor machine health in real-time. The data is analyzed on the manufacturing premises and analytics results are uploaded to centralized cloud data centers for reporting or further analysis.
EC has also been embraced by the retail sector. For example, retailers such as Nike and Walmart are increasingly using IoT sensors to track and analyze in-store customer behavior on premise. Behind the scenes, retailers use EC-enabled tools to track and manage inventory in real time, collecting powerful insights that can lead to better logistical practices down the line.
According to Gartner, though less than 10% of enterprise-generated data is created and processed at the edge as of now, that number is expected to increase to 75% by 2025. Market intelligence leader MarketsandMarkets also projects that the global edge computing market will grow from $3.6 billion in 2020 to $15.7 billion by 2025, at a CAGR of 34.1%.
“Organizations that have embarked on a digital business journey have realized that a more decentralized approach is required to address digital business infrastructure requirements,” says Santhosh Rao, senior research director at Gartner noted in a 2018 report. “As the volume and velocity of data increases, so too does the inefficiency of streaming all this information to a cloud or data center for processing.”
Data Monetization
Data and analytics are rapidly evolving from strategic assets to tangible financial assets. As companies look to both increase competitive advantage and identify/exploit now revenue streams, data monetization - the use of data to obtain quantifiable economic benefit - schemes are becoming increasingly common.
For example, car companies such as Ford and Tesla are exploring new ways to monetize driver data from using it to create use-based insurance companies to developing new, advanced safety systems to enhance the driving experience.
However, automakers are far from alone when it comes to identifying new ways to capitalize off of internal data. Media companies, financial services organizations and many more are upping their data monetization efforts and investments. In fact, according to recent industry studies, the data monetization market is expected to grow from $2.3 billion in 2020 to $6.1 billion by 2025 driven by increases in the volume of data generated and lower cost of data storage.
Reimagining the Road to AI
The race towards applied AI is officially on and, in 2021, we can certainly expect to see more organization develop new and exciting AI applications.
However, the past year has also proved to be a huge wakeup call for organizations when it comes to fully comprehending the current reality of AI vs. the hype. In between chip shortages and increased public scrutiny on the ethics of AI not to mention the low success rate of AI initiatives (at least when it comes to bottom-line impact), many companies are pulling back and rethinking their approach to AI development.
Google, for example, is completely reorganizing its AI team after confronting a number of ethics related AI scandals over the past year. This does not mean these companies are planning to reduce their AI spend or focus, but rather are realizing they must “tend their gardens” first in order to ensure they are well positioned to incorporate real, profitable AI applications in the near-future.
For many companies this will involve optimizing their data to AI pipeline by fortifying enterprise data and analytics infrastructure as well as reducing technical debt. For others it will mean working to develop more efficient and cost-effective ways of producing AIs. Regardless, as of now, AI is no longer a theoretical goal, but one companies are actively pursuing, albeit over the long-term.